Using high-resolution remote sensing and machine learning, researchers detected forest degradation, and found that forests lose 35% of carbon after fires
The Science
Forest degradation through fires and logging are widespread in the Amazon. Forest degradation changes the forest structure, but it is difficult to detect from space. A team of researchers used commercial satellites with very high resolution, and developed a machine learning system to automatically distinguish intact forests from logged or burned forests. They also used laser sensors on an aircraft to calculate how much carbon forests lose when they are degraded. To get the most precise impact of forest degradation on carbon stocks, the team considered that both their classification and their carbon stocks have uncertainties.
The Impact
The research team found that their machine learning method distinguishes degraded forests from intact forests in 86% of the cases. Sometimes the machine learning approach confuses logged forests with intact forests, but it is very good at identifying burnt areas. The team found that logged forests have almost the same amount of carbon as intact forests. However, forest fires can reduce the amount of carbon by 35%. The team also found that they needed to account for the confusion of the machine learning classification of forest degradation to correctly attribute carbon losses to forest degradation classes.
Summary
Forest degradation from logging and fires impacts large areas of tropical forests. However, the impact of degradation on carbon stocks remains uncertain because degradation is difficult to detect. This research used high resolution images from PlanetScope and produced a series of metrics that described the forest canopy texture. These metrics were then used to train a machine learning classifier that calculated the probability of forests being intact, burned or logged. The team also used biomass estimates from airborne lidar to calculate the impact of forest degradation on carbon stocks.
The classification approach has an accuracy between 0.69 and 0.93, depending on the site. This study found that changes in carbon stocks due to logging were small, but burned forests store 35% less carbon than intact forests. The team expected and found that uncertainty in carbon losses due to degradation increases when they account for the uncertainty in classification. However, they found that ignoring classification uncertainty could underestimate the impact of degradation on carbon stocks.
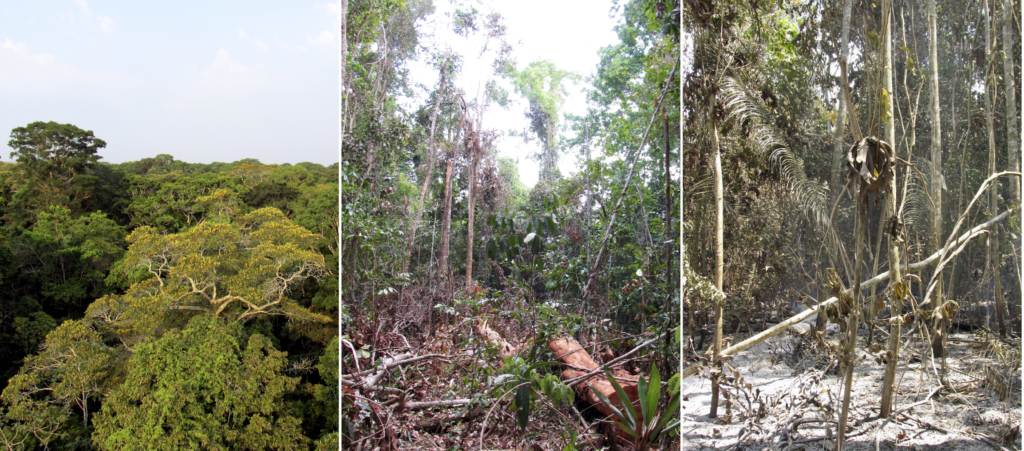
Contact: Ekena Rangel Pinagé, College of Forestry, Oregon State University (rangelpe@oregonstate.edu)
Marcos Longo, Lawrence Berkeley National Laboratory, (mlongo@lbl.gov)
Funding
This research was funded by the NASA Land Cover and Land Use Change Program, and by the Next Generation Ecosystem Experiments-Tropics, funded by the U.S. Department of Energy, Office of Science, Office of Biological and Environmental Research. The research carried out at the Jet Propulsion Laboratory, California Institute of Technology, was under a contract with the National Aeronautics and Space Administration.
Publication: Pinagé, E. R., M. Keller, C. P. Peck, M. Longo, P. Duffy, and O. Csillik, 2023: Effects of forest degradation classification on the uncertainty of aboveground carbon estimates in the Amazon. Carbon Balance and Management, 18 (1), 2, doi:10.1186/s13021-023-00221-5.