Study shows that reliable biomass estimates must include predictors that express inherent variations in tree species architecture.
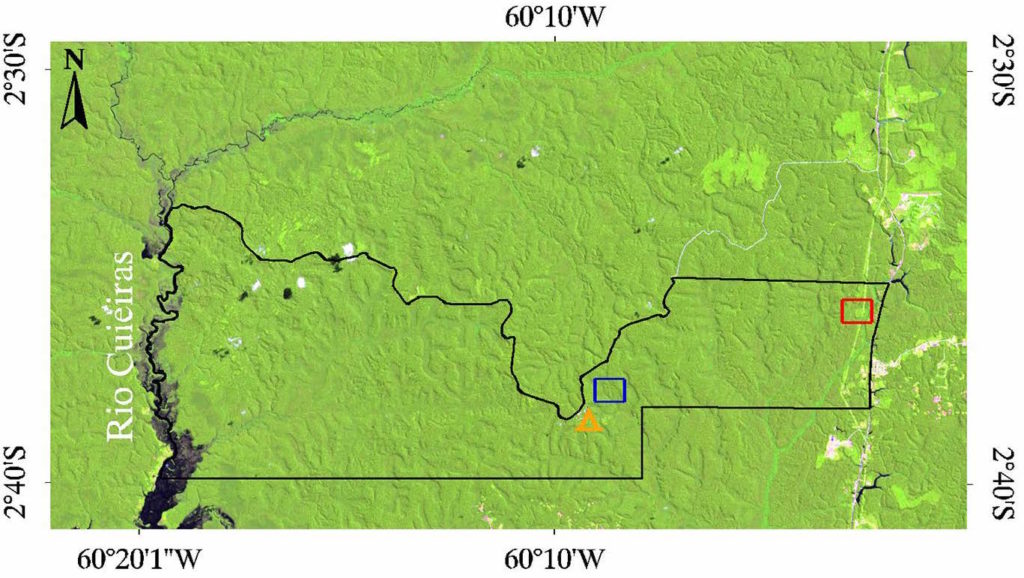
The Science
The hyper-diversity of tropical forests makes it difficult to predict their aboveground biomass levels based on biomass models that generalize across species. In a recent study, researchers employed a virtual forest approach using extensive field data to estimate biomass levels in the central Amazon.
The Impact
Due to the highly heterogenous nature of old-growth forests in structure and species composition, this study found that generic global or pantropical biomass estimation models can lead to strong biases.
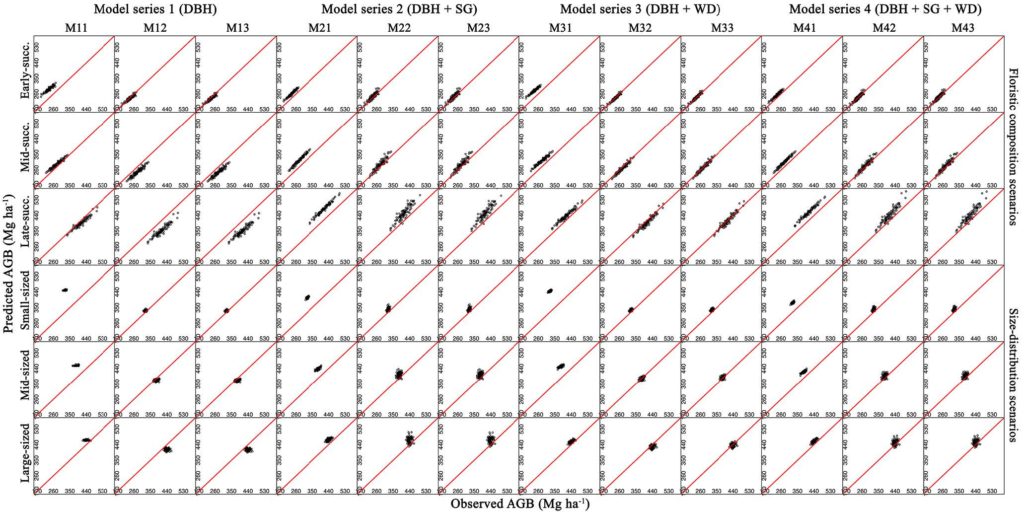
Summary
Old-growth forests are subject to substantial changes in structure and species composition due to the intensification of human activities, gradual climate change, and extreme weather events. Trees store circa 90% of the total aboveground biomass (AGB) in tropical forests, and precise tree biomass estimation models are crucial for management and conservation. In the central Amazon, predicting AGB at large spatial scales is a challenging task due to the heterogeneity of successional stages, high tree species diversity, and inherent variations in tree allometry and architecture. The researchers parameterized generic AGB estimation models applicable across species and a wide range of structural and compositional variation related to species sorting into height layers as well as frequent natural disturbances. They used 727 trees from 101 genera and at least 135 species harvested in a contiguous forest near Manaus, Brazil. Sampling from this dataset, the researchers assembled six scenarios designed to span existing gradients in floristic composition and size distribution to select models that best predict AGB at the landscape level across successional gradients. They found that good individual tree model fits do not necessarily translate into reliable AGB predictions at the landscape level. Predicting biomass correctly at the landscape level in hyperdiverse and structurally complex tropical forests requires the inclusion of predictors that express inherent variations in species architecture. Reliable biomass assessments for the Amazon basin still depend on the collection of allometric data at the local and regional scales and forest inventories including species-specific attributes, which are often unavailable or estimated imprecisely in most regions.
PI Contacts: Robinson Negron-Juarez, robinson.inj@lbl.gov and Jeffrey Q. Chambers, jchambers@lbl.gov
Publication
Magnabosco Marra, D., et al. “Predicting biomass of hyperdiverse and structurally complex central Amazonian forests: A virtual approach using extensive field data.” Biogeosciences 13, 1553–70 (2016). [DOI:10.5194/bg-13-1553-2016]. (Reference link)